Introduction
Airlines keep people around the globe connected and are a vital part of the aerospace industry. Global business and tourism wouldn’t be the same without the commercial aviation industry. It is the backbone of the modern world. However, air travel is still susceptible to disruptions from weather, ageing infrastructure, maintenance issues, congested airspaces, and suboptimal operational efficiencies. For instance, in 2022, U.S. passenger airlines’ average cost of aircraft block time was $101.18 per minute, leading to billions in additional expenses annually. Refer to the infographic below for more details on the diverse causes of aircraft delays. The FAA estimates these flight disruptions cost about $33 billion in the U.S. alone.light disruptions cost about $33 billion in the U.S. alone. Meanwhile, the demand for air travel is increasing, with the International Air Transport Association (IATA) projecting over 4 billion passengers by 2024, [surpassing pre-COVID-19 levels](https://www.iata.org/en/pressroom/2022-releases/2022-03-01-01).
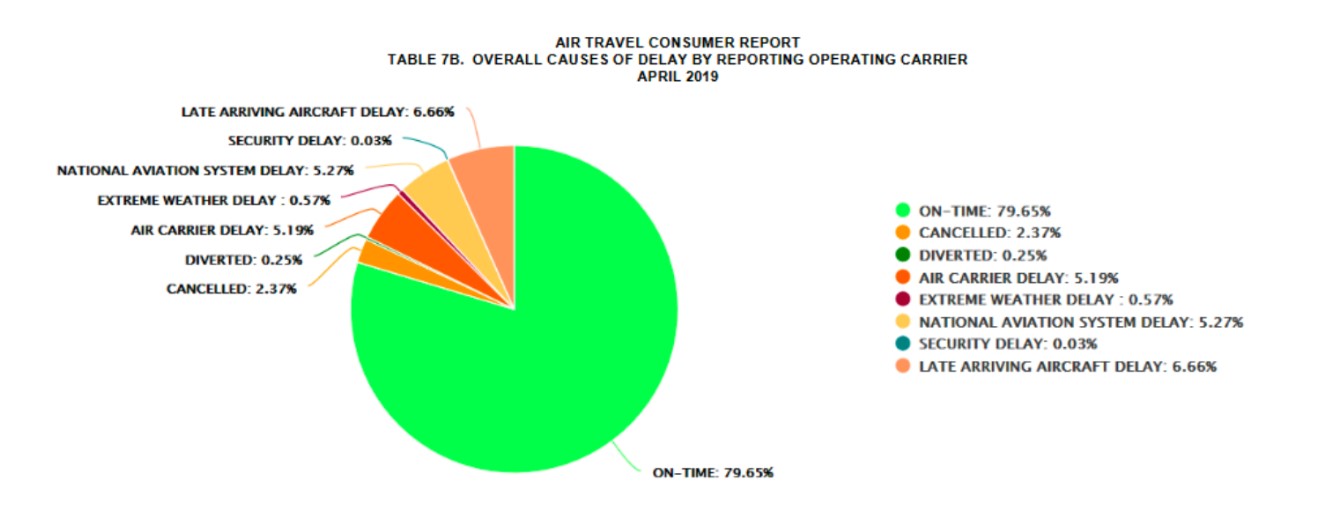
Artificial intelligence (AI) can address the pressing challenges facing aviation and, more broadly, the larger aerospace industry. AI can assist with everything from aircraft design to predictive maintenance and automated flight controls to enhance aerospace quality, safety, sustainability, and reliability.
In this article, we explore various AI applications in aerospace, focusing on how generative AI, computer vision, and IoT enhance efficiency and precision. We also examine the challenges associated with integrating AI into this complex sector, particularly regarding safety and regulatory compliance. Let’s dive in!
AI in Aircraft Design and Manufacturing
Designing an aircraft is a highly intricate process that involves many time-consuming and iterative steps. A designer would require extensive knowledge and expertise to work on such a project. This can be both costly and resource-intensive. This is where AI can jump in and help manufacturers and product designers cut costs without compromising quality or safety.
Generative AI in Aircraft Design
A branch of AI called generative AI uses generative design algorithms to generate, test, and refine aircraft component designs iteratively. These algorithms aim to meet specified objectives around strength, weight, manufacturability, and cost.
Airbus has been using generative AI to develop lightweight partition designs for aircraft cabins. This technology considers various factors such as structural load requirements, spacing needs, and weight limits. Through computational evolution, generative AI efficiently explores design options to create partition architectures optimised for both strength and lightness, ultimately contributing to reduced fuel consumption.
A great example is the ‘bionic partition’ developed for the Airbus A320. This partition is a result of a collaboration between Airbus and Autodesk. The bionic partition weighs 45% less than traditional designs. When applied across the entire cabin of the A320 fleet, Airbus estimates a possible reduction of up to 465,000 metric tonnes of CO2 emissions annually.
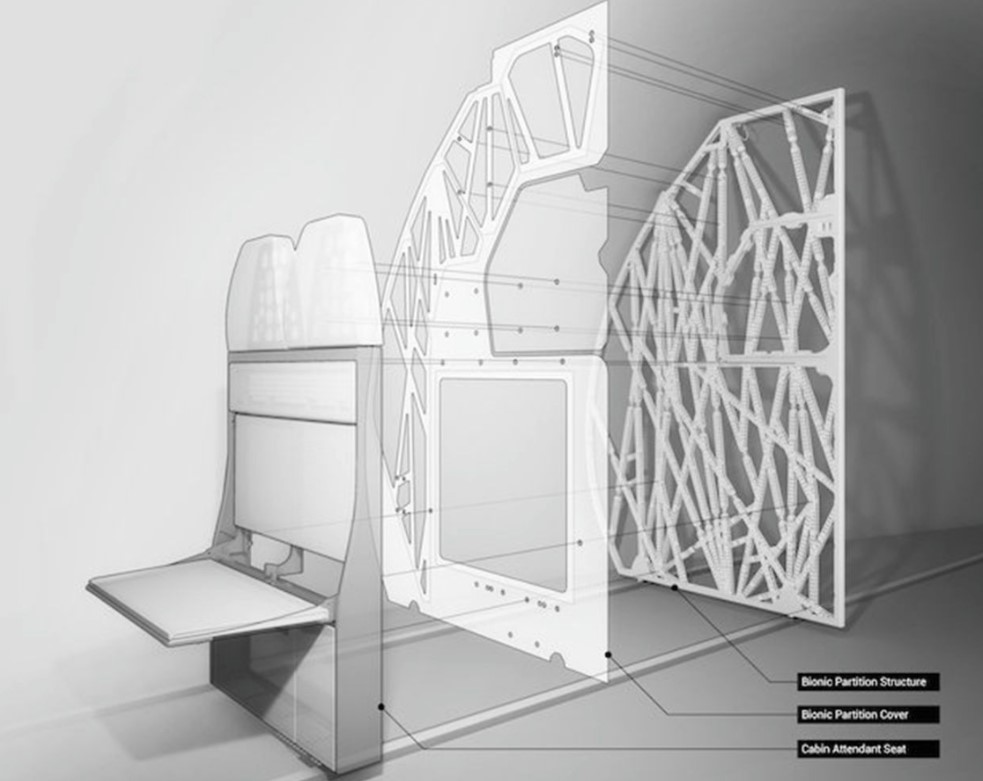
Boeing is also actively using generative AI models in its aircraft design processes. They are exploring innovative ways to optimise aircraft design, including passenger cabin layouts. During a panel discussion at the Sustainable Aerospace Together Forum, Boeing’s Chief Technology Officer, Todd Citron, highlighted the company’s use of generative AI models in aircraft design.This technology is not just a futuristic concept but a present reality.
Now that we’ve looked at how generative AI aids in designing aircraft components, let’s understand how AI can assist in prototyping and testing to see the practicality of bringing these designs to life.
AI in Prototyping and Testing
When it comes to prototypes and testing, AI-driven simulations have several advantages. These simulations predict how a prototype will perform under various conditions without actually having to build or physically test the prototype. This predictive capability allows engineers to identify potential problems early in the development cycle and make necessary changes at a much earlier stage. The result is a more efficient development process.
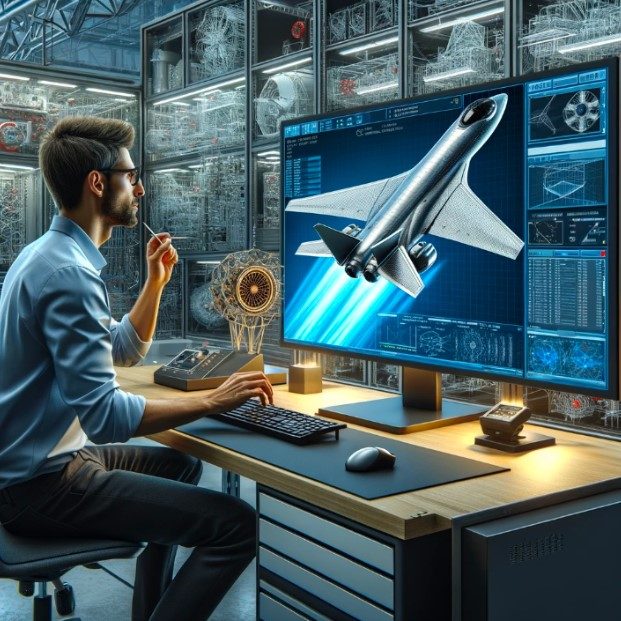
One important factor contributing to the efficiency of AI-driven simulations is the utilisation of GPU acceleration for faster computing. GPUs are particularly good at handling multiple tasks simultaneously through parallel processing. This parallel processing capability allows these simulations to conduct an impressive number of design evaluations compared to traditional methods.
Once a design prototype is tested and ready for production, the focus shifts to the manufacturing process, where AI can help ensure precision and quality.
Computer Vision in Aerospace Manufacturing
In aerospace manufacturing, a subfield of AI known as computer vision enables precise quality inspection and tracking of aircraft components throughout the assembly process. Boeing uses automated optical scanning and dimension analysis to quality-check sections of aircraft wings versus engineering drawings during fabrication.This AI-powered process validates component size, shape, alignment, and drill precision for different features on a typical wing, saving substantial manual effort.
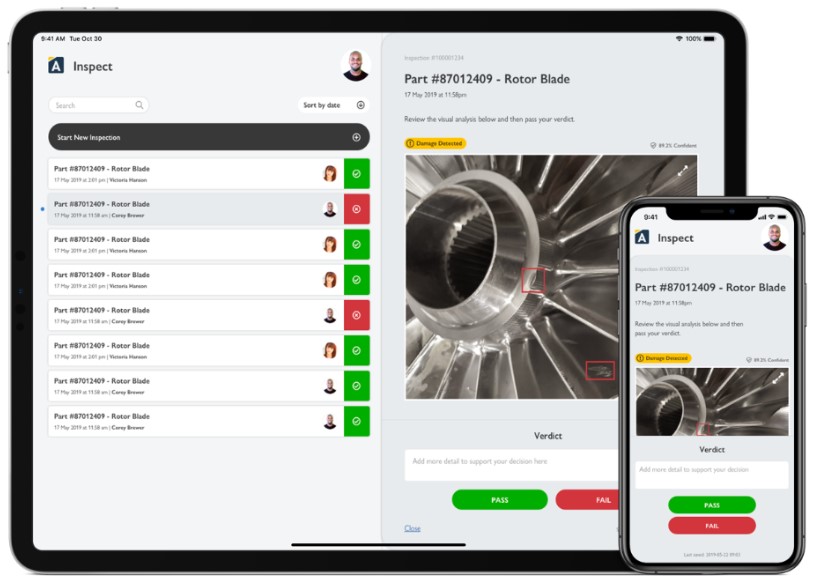
Computer vision can also facilitate monitoring critical aircraft system assembly more accurately than human supervision. Augmented reality headsets, equipped with computer vision, assist technicians by visually projecting detailed, step-by-step instructions overlaid on the assembled components. Before authorising process sign-off, the AI confirms proper assembly by comparing live views versus digital models. Through these innovations in design, production monitoring, quality assurance, and guided assembly, AI aims to transform aerospace manufacturing quality and productivity.
AI in Flight Operations and Control
Fight planning and air traffic management are being redefined by AI algorithms that can parse weather forecasts, equipment availability, crew scheduling, and other data to optimise routing plans. Qantas, for instance, has reported significant efficiency improvements in flight planning with its AI system, Constellation, developed in partnership with The Australian Centre for Field Robotics at The University of Sydney. This advanced 4D system analyses factors such as lateral and altitude positions, speed, and weather data to reduce fuel usage and CO2 emissions. The system’s adoption by Qantas is anticipated to result in considerable annual savings — approximately $20 million in fuel costs. Additionally, it is expected to decrease CO2 emissions by 50 million kilogrammes each year.
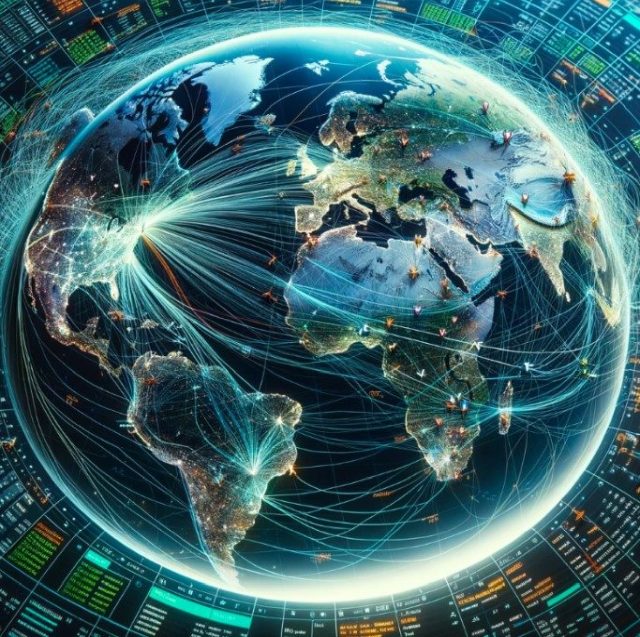
With respect to air traffic control, predictive analytics applied to historical traffic patterns, flight schedules, and aircraft tracking data enable the identification of future congestion “hotspots.” This technology allows controllers to proactively reroute flights around anticipated delays before they occur rather than addressing problems reactively mid-flight. As global air traffic gradually returns to pre-pandemic levels, AI automation will be crucial in managing this increasing complexity.
Predictive Maintenance through AI
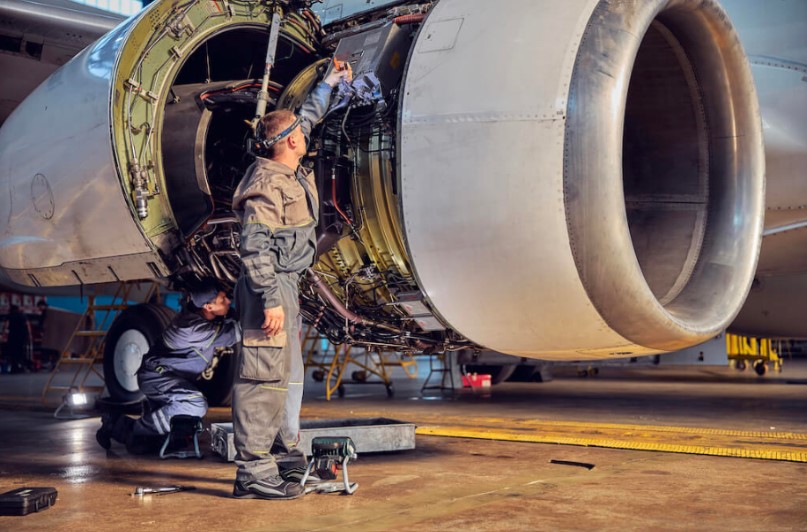
The aerospace industry is increasingly turning to AI for predictive maintenance. By leveraging IoT edge computing and AI analytics, airlines can monitor aircraft subsystems in real-time, predicting and preventing potential failures before they occur.
IoT sensors are crucial in this setup. They are installed throughout the aircraft to collect real-time data on parameters such as engine vibrations, heat, RPMs, and fuel flow. This continuous data stream provides a comprehensive view of the aircraft’s health. Instead of sending all sensor data to a central server, edge computing allows data processing to occur directly on the aircraft. This reduces latency, enables faster decision-making, and minimises the need for constant data transmission, which is critical in environments where connectivity might be intermittent or limited.
However, the real power of predictive maintenance lies in AI analytics. Machine learning algorithms analyse vast amounts of sensor data, comparing it with historical patterns and performance benchmarks. By doing so, AI models can detect even slight deviations that might indicate potential issues long before they become serious problems.
The advantages of using AI and IoT edge computing for predictive maintenance are that airlines can anticipate maintenance needs, reducing unexpected downtime and prolonging the life of aircraft components. This proactive approach to maintenance not only saves costs but also enhances safety. Additionally, it allows for more efficient scheduling of maintenance work, avoiding disruptions to flight schedules and improving overall operational efficiency.
AI in Enhancing Passenger Experience
AI can also help enhance passenger experience through inflight entertainment systems. Honeywell and Thales are incorporating AI content recommendation engines to provide personalised movie, news, and destination suggestions tailored to individual passenger preferences and travel details. Seatback touchscreens may also eventually host AI-powered conversational travel assistants like Siri, Alexa, or Google Voice. These virtual assistants could answer passenger questions and facilitate requests around the clock.
Passenger experiences aren’t limited to the flight itself. Even ground operations can be improved by AI. Computer vision can be used in check-in, security, and boarding processes to enhance passenger facilitation. Narita and Haneda airports in Japan have implemented facial recognition systems for a more efficient and touchless experience. This “Face Express” system allows passengers to go through baggage check-in, security, and boarding without the need to show passports or boarding passes, all verified through facial recognition.
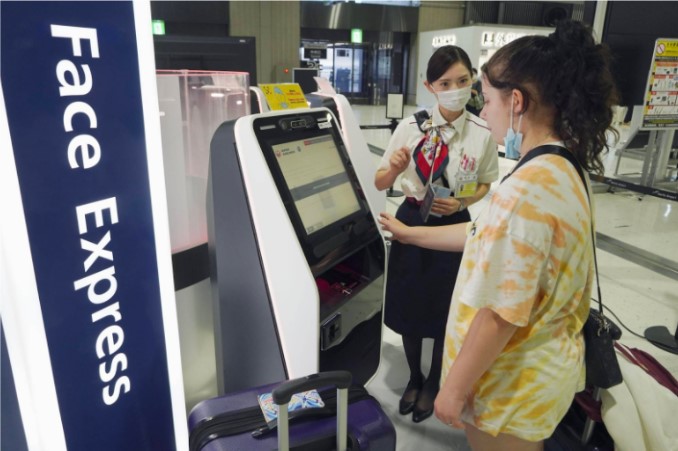
Challenges in Implementing AI in Aerospace
Despite promising capabilities in design, predictive maintenance, and passenger experience, adopting AI in mission-critical aerospace operations faces trust and governance barriers. Can generative algorithms reliably design components sustaining passenger safety in extreme edge-case situations? What risks are associated with bias in training data influencing air traffic management decisions?
To ensure safety in aerospace systems, rigorously testing and validating AI technologies is crucial. This involves simulations of various scenarios outside normal operating conditions to prepare for rare but critical safety issues. To certify autonomous aerospace systems for widespread use, they must demonstrate reliable performance through extensive simulated testing, covering billions of test miles. This thorough testing is key to deploying these systems safely on a large scale.
Meanwhile, ambiguous regulations around autonomous flight operations complicate certification and operational approvals. Today’s policies struggle to handle emerging technologies, from passenger drones to single-pilot cargo jets. As AI technology in aircraft rapidly advances, there’s a risk that regulations and governance might not keep pace. This could happen before we fully address all safety concerns and reliability questions related to these advanced AI systems. Therefore, successfully adopting transformative AI aerospace systems requires collaboratively addressing these concerns early across stakeholders spanning technologists, aircraft manufacturers, airlines and operators, infrastructure providers like airports and Air Traffic Control, policymakers, plus the travelling public.
What We Can Offer as TechnoLynx
At TechnoLynx, we specialise in providing AI solutions tailored specifically to your requirements. Our focus is on integrating AI in ways that address stakeholders’ unique challenges and requirements. This includes customising AI applications for industries like aerospace. Our AI solutions are not just technically advanced but are also practical and relevant to the specific needs of each project. We believe that every AI endeavour requires a unique approach.
Our expertise in computer vision, generative AI, GPU acceleration, and IoT edge computing can help you explore many possibilities. We aim to push the boundaries of innovation while ensuring adherence to rigorous safety standards. For more information, feel free to contact us.
Conclusion
AI enhances various aspects of the aerospace industry, from aircraft design and manufacturing to flight operations and passenger experiences. However, integrating AI in aerospace also presents challenges, especially regarding safety, trust, and fast-paced technological advancements and regulatory frameworks. Addressing these challenges in the aerospace industry requires a collaborative approach among all stakeholders, including an AI solution provider that fully understands and addresses your concerns. At TechnoLynx, we specialise in offering customised AI solutions to navigate these challenges effectively, pushing the boundaries of innovation while ensuring safety and compliance.
Sources for the images:
-
All Things On Time Performance (2020) Flight Delays in Numbers – Not Only Painful For Passengers.
-
Airbus (2016) Pioneering bionic 3D printing.
-
Bjerregaard, L. (2019) Boeing Selects AI Startup To Enhance Parts Inspections Aviation Week Network.
-
KYODO (2021) Narita and Haneda airports start wider use of facial recognition The Japan Times.
-
ToolSense (n.d.) Why Predictive Maintenance in Aviation Can Save Lives.