Introduction
Generative AI is a branch of artificial intelligence that can create fresh and unique content without human intervention. These impressive abilities include independently generating text, images, audio, video, and other multimedia materials after being taught using extensive datasets.
What sets generative AI apart from other AI systems is its capability to produce new and original artifacts and content by studying patterns and connections in its training data. Prominent generative AI models such as OpenAI’s GPT-3, which excels in text generation, and DALL·E 3, known for its image generation, have showcased the immense creative potential of this technology.
With each passing day, these models are becoming more sophisticated and easily accessible, making them essential tools for anyone looking to tap into the creative potential of AI. However, addressing concerns surrounding potential biases, misuse, and intellectual property implications is important as generative AI evolves.
This evolution is changing how we create content and transforming our methods of problem-solving and innovation in multiple sectors. As we enter this new era, our article will explore the profound effects of generative AI, its uses, and the ethical questions it raises in our ever-growing digital society.
Applications of Generative AI Across Industries
Generative AI is already beginning to transform major industries by automating, augmenting, and enhancing content creation pipelines. Let’s discuss some examples of how businesses are applying generative models.
Drug Discovery
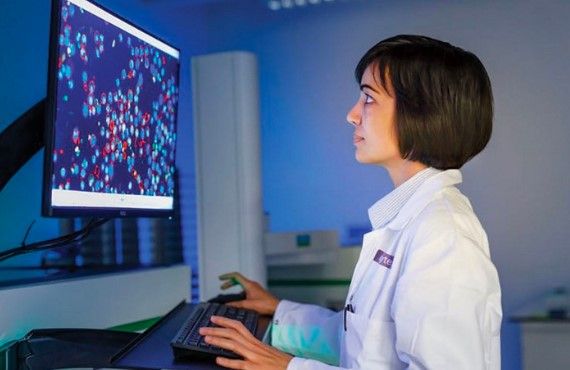
Generative AI can significantly accelerate the drug discovery and development process. Traditional drug discovery involves laborious trial-and-error experimental approaches to identify promising molecular compounds with desired pharmaceutical properties. This can take over a decade in some cases. AI-based generative models like Exscientia’s Allcyte can propose and evaluate billions of molecular structures to find candidates with high potential to become safe, effective drugs for targets like COVID-19.
Computer Programming
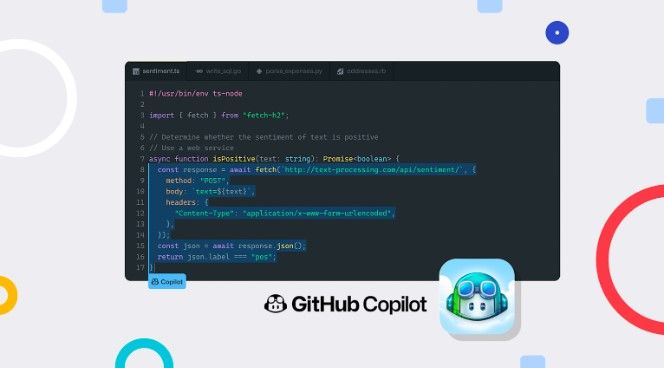
Generative AI is advancing in software development as well. Modern IDEs, such as GitHub Copilot, use generative models to suggest complete lines or even whole functions of code while programmers type. These suggestions are based on the context gathered from training on extensive codebases, which speeds up the development process by reducing repetitive and boilerplate coding tasks. AI-powered tools can also generate entire programs from natural language descriptions, helping to initiate new projects quickly. As these generative coding assistants evolve, they are expected to boost developer productivity significantly.
Fashion
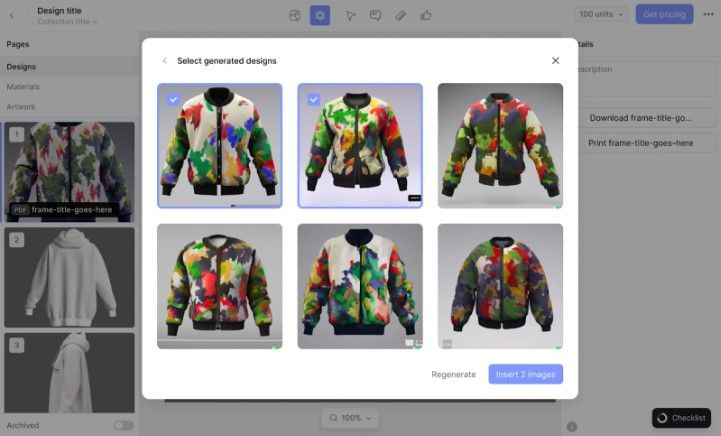
Generative AI models in the fashion industry, like those used by Adidas, can independently create customised clothing, footwear, and accessories. They tailor these items to individual body measurements, style preferences, current trends, and user-provided mood boards. Acting as an always-available fashion designer, generative AI rapidly prototypes unique designs. These designs can incorporate specific fabrics, colours, patterns, and shapes chosen by the user. This technology is transforming design workflows, enabling fast iterations and the mass customisation of apparel.
Music
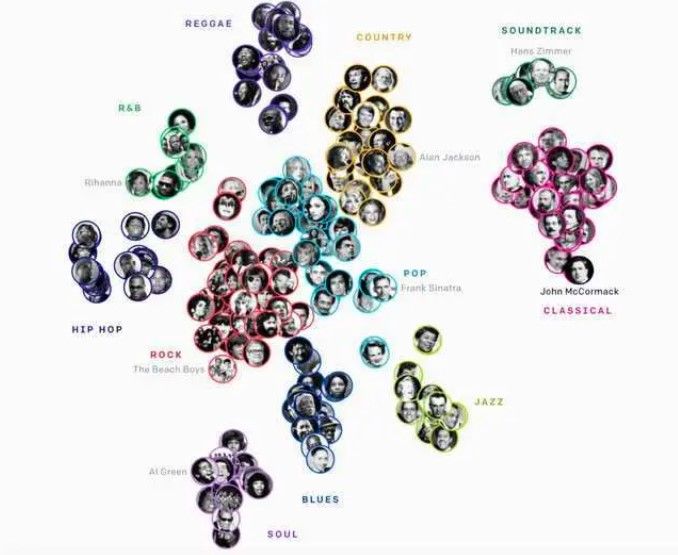
Innovative generative AI models such as Jukebox, MusicLM, and Riffusion are transforming procedural music generation. They autonomously produce original compositions, instrumentals, and music tailored to specific genres, moods, and instruments. Artists can use these tools to experiment with different melodic variations or to generate entire background scores. They can do this simply by specifying the desired length, style, and instruments. Models like Magenta’s NSynth also synthesise high-fidelity audio, not limited to music. It can reconstruct characteristics of previously unseen instruments. These advancements are democratising sound production, allowing even non-musicians to generate soundtracks from text prompts.
Visual Digital Art
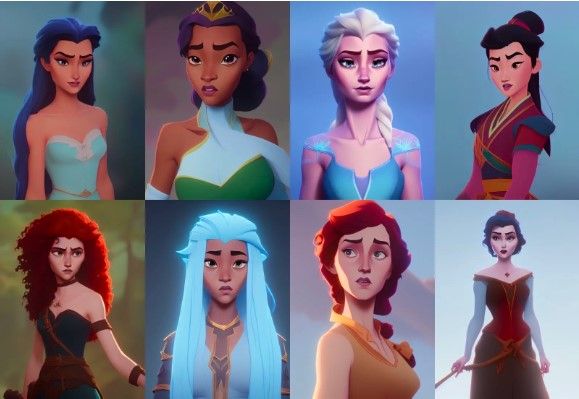
Generative art tools such as DALL-E and Stable Diffusion are transforming visual content creation. They enable users to convert imaginative concepts into digital art. For instance, one can create images like “teddy bears dancing in a futuristic nightclub” or “an astronaut riding a horse on Mars” from simple text prompts. Similarly, architects and designers use tools like Adobe’s Creative Cloud for the automatic generation of 3D interior renderings and floor plans. These match the desired aesthetics and layouts with ease.
Lead Generation
Lead generation is the lifeblood of growth and success in today’s highly competitive business landscape. It is the process of identifying and attracting potential customers or clients who have shown an interest in a product or service. Traditional lead generation methods often involve manual processes, cold calls, and mass marketing campaigns. However, with the advent of Generative AI, lead generation has evolved significantly. Companies now leverage the best AI lead generation tools to automate and refine the process. AI-based lead generation tools utilise data-driven insights and predictive analytics to identify high-quality leads, tailor marketing efforts, and improve conversion rates. This transformative technology not only saves time and resources but also enables businesses to target their audience with greater precision, resulting in a more efficient and effective lead generation process.
Benefits of Generative AI
Generative AI offers significant advantages over traditional content creation methods. It accelerates iteration cycles, enabling creators to generate and refine ideas quickly. Personalisation is another strength of generative AI, allowing for content that caters specifically to individual users’ preferences, such as product recommendations, clothing designs, or music playlists.
Additionally, generative AI can save substantial cost and time by automating manual tasks and reducing the need for expensive prototyping and labour. With the ability to operate around the clock, AI facilitates a faster content development process. In essence, generative AI enhances human creativity with higher quality, variety, and efficiency in content production, marking a transformative shift in the industry. Businesses should consider its potential to revolutionise content creation.
Current Limitations and Challenges Facing Generative AI
Despite the promising capabilities and benefits discussed so far, some notable limitations hold generative AI back from reaching its full potential.
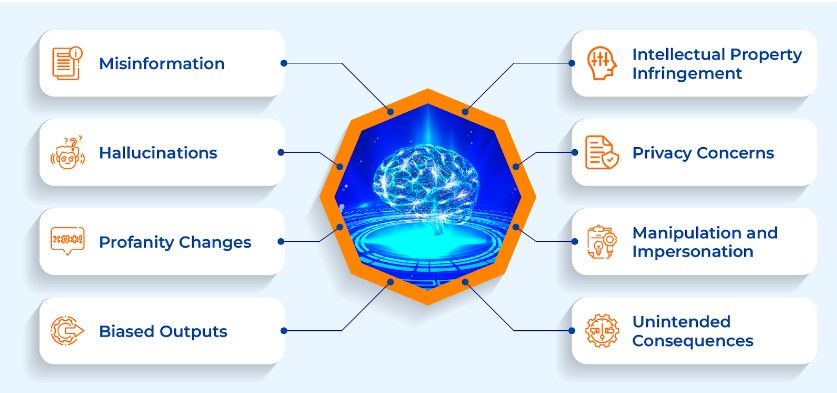
These limitations do not diminish the technology’s promise but require thoughtful solutions. Addressing these challenges will need a multi-pronged approach. This should involve technical fixes, policies, and self-regulation. Researchers, developers, and governments must work together to guide the responsible development of generative AI as its capabilities expand. Keeping that in mind, let’s walk through the different challenges facing generative AI.
Training Data Biases
Like any deep learning system, generative AI models inherit the biases present in their training data. Models trained on biased datasets generate less desirable results overall. Addressing dataset biases and utilising techniques like data augmentation is critical to make generative models fair and representative. Recently, many models have been trained keeping this in mind and have seen wide acceptance.
Heavy Computational Requirements
The scale of datasets and model architectures required to train powerful generative AI systems entails massive computational resources for hardware, energy, and cloud computing access. Deploying these models can be expensive and environmentally taxing. Optimising generative models for efficiency and making them available through APIs helps expand access, but resources remain a barrier for many potential users.
Copyright and Ethical Concerns
The copyright status and ownership of AI-generated artifacts is still an open debate. Training datasets typically contain copyrighted works, adding legal ambiguity around model outputs. There are also concerns about how generative content could be misused to spread misinformation, forged imagery, or personalised spam at scale. Implementing mechanisms to detect fakes and holding users accountable will be vital to preventing harm as generative AI progresses.
Ongoing Developments Shaping The Future of Generative AI
The landscape of generative AI is changing quickly, thanks to the work of researchers in both business and academia. They’re finding ways to overcome current challenges and expand what AI can do. As these improvements build on one another, we can expect generative AI to become much more effective and avoid the issues we see today. Soon, we might have advanced AI assistants that can work with us on various projects, changing how we think about creativity and innovation.
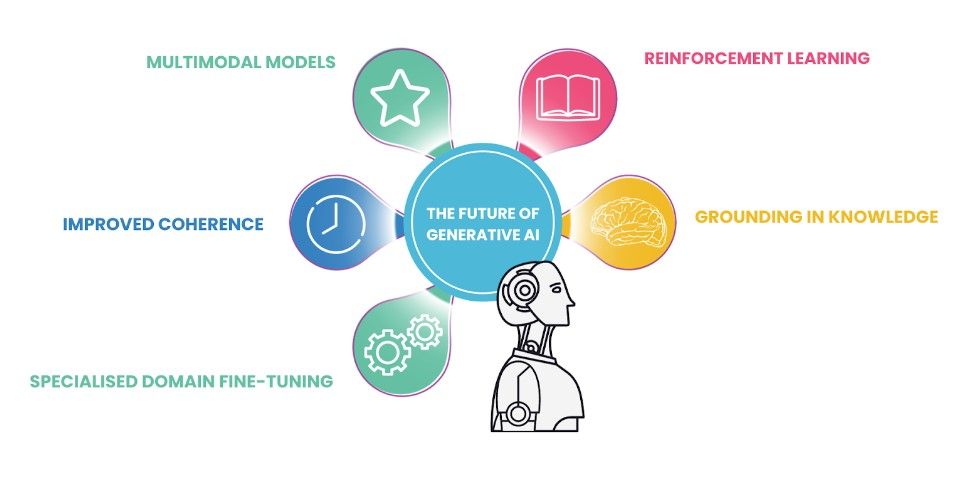
Here’s a look at some of the exciting developments guiding the future of generative AI.
Multimodal Models
Rather than focusing on a single output type like text or images, newer models aim to generate content and transition seamlessly across multiple mediums like text, image, audio, and video. For instance, Google’s Bard can describe images with captions and answer questions. Models with integrated reasoning across modalities promise more general and widely capable creative assistants.
Improved Coherence
Techniques such as chain-of-thought prompting lead generative models to create more logical and internally consistent outputs by providing explanatory context, not merely a few words. Models that are explicitly trained to grasp causality - understanding the relationships between causes and effects - also minimise non-sequiturs, which are statements that do not logically follow from what preceded them in the generated text. Additionally, using more structured workflows for image generation enhances the coherence of the visual outputs.
Specialised Domain Fine-Tuning
Pre-trained general-purpose models, such as GPT-3, can undergo further training on niche datasets. This additional training, related to a specific task or industry, refines their outputs. As a result, domain-specific models tailored to fields like biology, robotics, or law are created. These specialised models generate highly customised and relevant content.
Reinforcement Learning
Algorithms used for reinforcement learning reward generative models for producing desired outputs and provide feedback to guide their behaviour. This enables developers to align the models with human values and goals. Reinforcement learning holds the potential for diminishing biases and toxic output.
Grounding in Knowledge
Connecting generative models to external knowledge bases and equipping them with semantic reasoning and memory capabilities allow them to incorporate real-world facts and logic into content generation. This enhances accuracy and reduces the creation of unrealistic content.
The Business Potential of Generative AI Across Industries
Generative AI is quickly proving to be more than just a source of novelty in art and storytelling. In the business world, it’s unlocking new possibilities and driving value in several impactful ways.
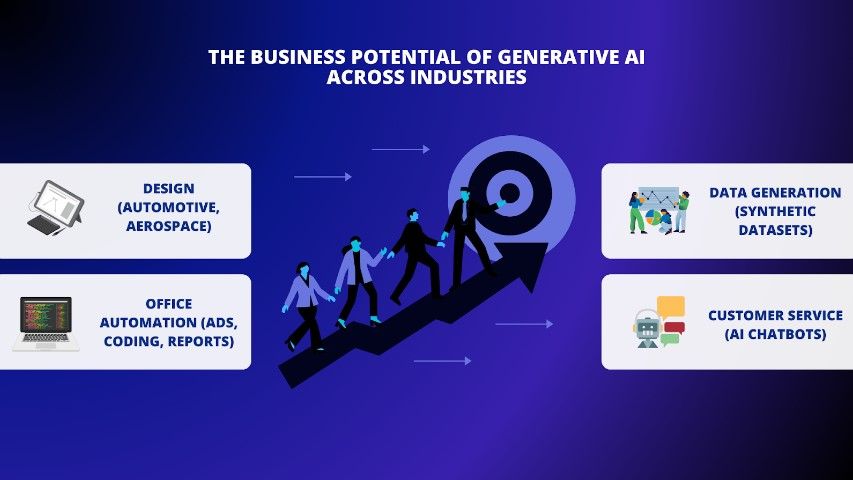
For instance, in industries like automotive and aerospace, companies are using AI to whip up design variations at lightning speed. This isn’t just about making things faster; it’s about creating stronger, lighter parts that could have taken months to design manually. And it’s not just planes and cars; everything from phones to pacemakers could be designed smarter and faster with AI.
Then there’s the day-to-day grind in offices. Imagine if routine tasks like making ads for different markets, coding the same kinds of functions, or digging through financial reports could be done at the click of a button. That’s where generative AI takes on these repetitive tasks and lets people focus on the bigger picture.
Data is another frontier where AI is a game-changer. In fields where accurate, usable data is a rare commodity, AI can create synthetic datasets that look and act like the real thing, which is a big deal for training other AI systems, whether they’re helping choose your next favourite movie or making sure a self-driving car can handle the road.
And let’s not forget about customer service. AI chatbots are becoming sophisticated enough to handle customer questions and issues, providing helpful support without needing a human on the other end of the chat. This can make getting help faster and easier for customers and more cost-effective for businesses.
In short, generative AI is reshaping the business landscape, offering smarter design tools, efficient workflows, quality data, and improved customer interactions. It’s an exciting time for businesses willing to embrace these new tools.
Conclusion
Generative AI is not just a tool for innovation but a gateway to transforming multiple industries. The possibilities are endless, from enhancing creativity in digital arts to redefining methodologies in medical research and engineering design.
However, navigating the complexities of Generative AI requires expertise and tailored solutions. This is where TechnoLynx steps in. Our cutting-edge software R&D consultancy specialises in custom Generative AI modelling, offering unique solutions catering to your business needs.
From creating next-generation cosmetics treatments to designing immersive gaming experiences and optimising automotive manufacturing, our expertise is as varied as it is deep. Our portfolio includes transformative projects like AI-based medical imagery software, showcasing our capability to handle complex and innovative challenges. This diversity in application, coupled with our commitment to custom solutions and advanced technical skills in areas such as auto-encoders, GANs, and transformer models, positions TechnoLynx as a leader in the field of Generative AI.
Sources for the images:
Leskin, P. (2020). This AI is creating some surprisingly good bops-based on music by Katy Perry and Kanye West. Business Insider India. Mullin, R. (2021). Exscientia enhances AI with acquisition of Allcyte. C&EN. Available here. Otemuyiwa, P. (2021). Cloudinary. Available here. Singh, T. (2023). SearchUnify. Available here. Smith. S. M. (2022). IEEE Spectrum. Available here.