The Illusion of Opportunity
I would definitely be very late to come and sing the praise of all the recent advancements in AI-as-a-service, mentioning things from the conscious-according-to-some GPT-variants to the best Midjourney fakes, so it’s a good thing that I didn’t plan to. For starters, yes, it is indeed true that, in many ways, the combination of iterative R&D and unrivalled budgets to train massive models led to unexpected results. In principle, I will present no arguments against the underlying technologies; my problem is the business model.
Running the R&D consultancy TechnoLynx, we are getting plenty of inbound requests asking our opinion and help on building solutions on top of existing AI-as-a-service systems, and I’ve had to explain their limitation so frequently that I now believe that due to the prevalence of fledgling AI startups attempting to capitalise and commercialise said services, it might be of public interest to talk a bit about the downsides too.
The story I frequently hear goes along these lines: “AI became a game of giants, who are very quick and very competitive in rolling out rivalling AI solutions as services, which are, on the one hand, great enablers for startups to build an eco-system based on them, but they have also elevated the bar on core AI so high, that it became pointless even to try to invest in that space anymore. The emergence of AI-as-a-service (SaaS wrappings) is a sign of the maturity of the technology, and this is how things will be from now on. To each their own, burn your data science books and clear your whiteboards! Let’s all go and play with a bit of prompt engineering instead!” Well, rapid prototyping using Lego blocks certainly has an appeal, but let me put my mouth where my money is.
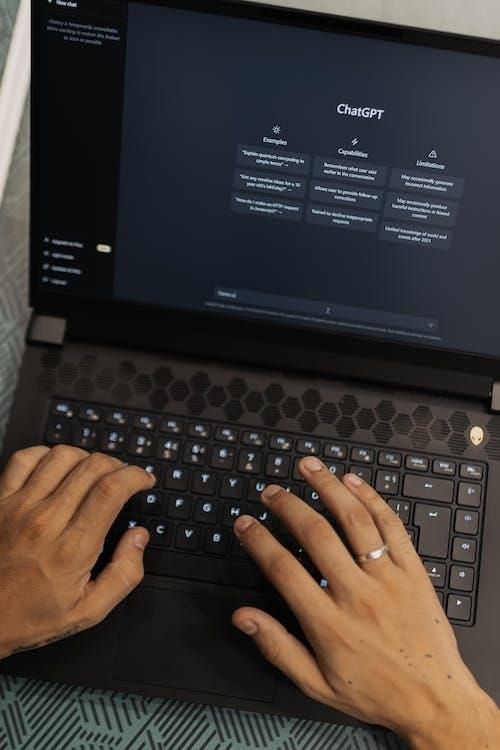
1) Lack of Quality Control
My first practical concern is the limitations on quality control. Most tech-business owners would sleep better knowing that if something goes haywire, their team has the means to fix things, as much as bug fixing was a thing in the good old days of the Software 1.0 world. Albeit we often hear the argument that AI models are black boxes that we cannot possibly decipher anyway (to some extent, this is true), there are still differences between a black box that you don’t completely understand, but you can communicate with and train it incrementally better, and a Supermassive Black Hole of the Unknown put behind a convenient API by a 3rd party. In general, it should be possible to some extent alleviate this problem by having external, custom supervisory networks or with ideas like how ControlNet operates, but the natural way of working with deep learning models would, at the very least, enable unfettered gradient flow, which is not currently available. Hence, the means for quality control are barely existing.
Working under such conditions creates such a dependency on the underlying service that, in practice, relegates this current generation of fresh AI entrepreneurs to operate merely as salespeople for Sam Altman. It might have been your plan all along, but then why didn’t you just apply for a job at OpenAI?
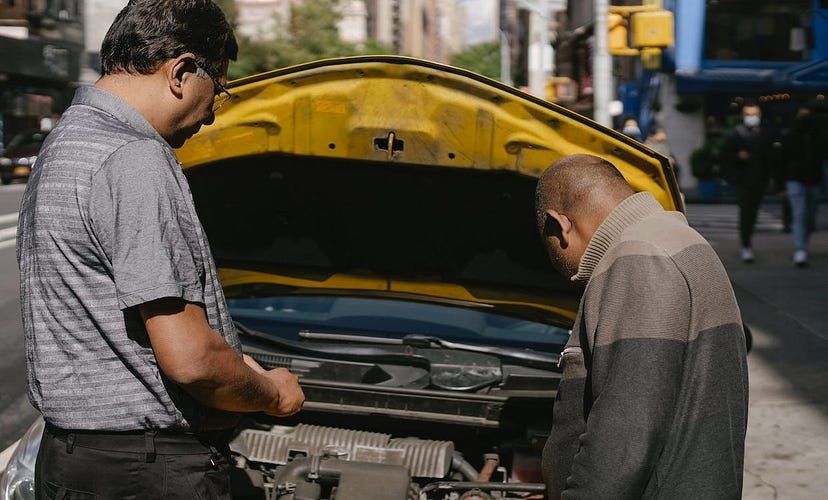
2) Limitations of Customisation and Differentiation
Almost the same argument as just above, but not precisely, as there are two further sub-cases here. Most AI-as-a-service systems like ChatGPT already offer support to some extent of customisation, whether it be via refinement training or context-feeding. At present, context size and the general behaviour of forgetting it over time of use may be a practical issue, whilst for refinement training, whilst it is a valid strategy, the effect compared to vast amounts of pre-training may very well be more limited than expected.
Having said that, the sub-cases are as follows, from a practical point of view: the options on offer for customisation may prove insufficient, and should that be the case, as a user, you would have no way to expand upon them forcefully. If you could customise enough, good for you, but if not, wait for a few months/years to be at the mercy of your service provider before they enable you.
On the other hand, customisation and ease of use may be, on the contrary, super-accessible. As we see the story with prompt engineering, it is more like a game of “-Oh, but the AI cannot solve this problem! — Yes, it can; you just need to ask it the right way! — How do I learn that? — You don’t have to, just use this kit PE + AI, and it does that for you!”, ultimately leading to something accessible. Yes, you figured it out: if you could use it efficiently, so could others, and you just witnessed your window of opportunity getting closed.
3) Privacy Issues and Ethical Concerns
Based on the previous paragraph, let’s assume that refinement training, or even some kind of online training, is available for your choice of AI-as-a-service. So far, it was crystal clear to everyone that the primary enabler and differentiator in the AI race is access to better quality and more diverse data, preferably from some live source you control. Here comes AI-as-a-service, and all of a sudden, nobody minds building such sources as part of the eco-system-building exercise and handing data over to their preferred AI-as-a-service provider!
Let me be very clear: nothing has changed, and data is still king, but you may not be for long unless you are very careful about whom you trust with it.
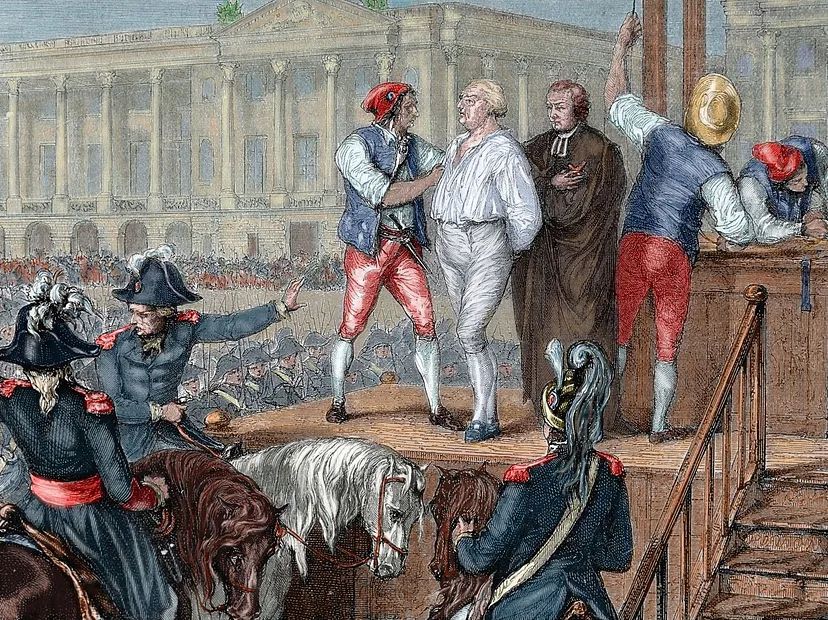
Unfortunately, the same applies not only to your data but also to the vendor’s training data. Behind the API firewall, you will hardly ever know what kind of data was used for training, if it was ethically sourced with appropriate consent, or if it was representative enough of all demographics. E.g. in the case of ChatGPT, most nations by now are pretty aware of the massive bias towards the corpus of the Anglosphere. There is no reason to believe the situation would improve much in general.
Not to mention that lacking oversight of the complete training process and data also means that testing may be undermined by having an overlap between training and test data. The chance of this might be insignificant for large language models of general purpose. Still, for LLM specialisations targeting specialist topics (so pretty much any actionable idea with business value in the space), the chances of overlap are far higher, given the limitations of total corpus size.
How Can I Succeed Then?
For starters, don’t try and trust your luck so much. You will not find low-hanging fruits. You will need to work hard, and working hard in this space means putting effort into proper research and development and owning the technology you rely on. On the other hand, don’t believe what others are telling you. The barrier to entry is not as crazy high as more prominent companies want you to feel. Quite the contrary, the progress on the R&D side is entirely incremental in nature, and the effort to publish recent results as whitepapers and sometimes as open databases is still very much alive. The baseline technology level available is solid in general. The only thing that requires tremendous resources is the ability to show a momentary fickle of progress never seen before — and even that advantage seems to have a very short half-life in practice. Core R&D on AI is not a finished business, and there is no proof that actual breakthroughs could only come from big players. The game is open for startups and organic SMEs alike. Indeed, building an engineering team capable of doing relevant research whilst also developing practically usable software is not easy. Still, for all of you aiming for it, TechnoLynx would be happy to listen to your ambitious ideas and chart a way forward together, with fundamental R&D over playing with Lego blocks. There is nothing wrong with Lego blocks, either. I also used to play a lot with them, up until elementary school.
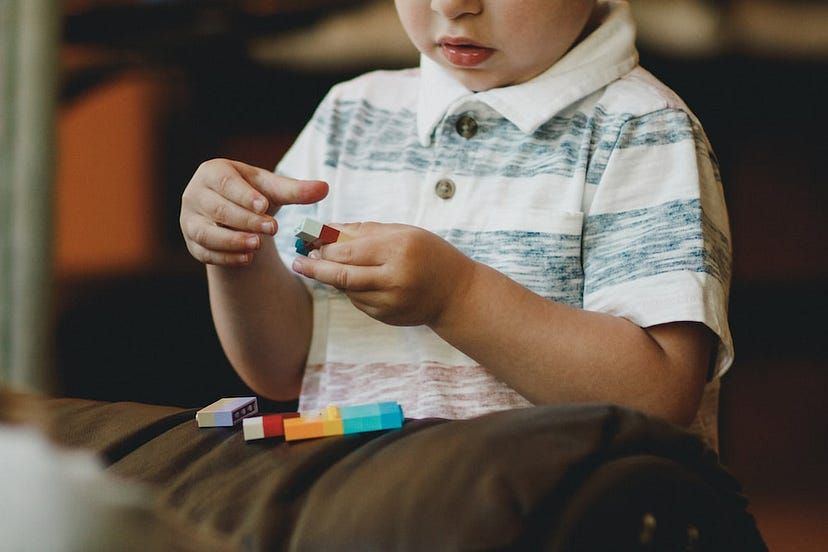