Introduction
Self-driving cars used to be science fiction, but with the help of AI, autonomous vehicles are becoming a reality. In fact, the autonomous vehicle market space is expected to reach a value of USD 93.31 billion by 2028, growing at a CAGR of 22.75% during the forecast period (2023-2028). This remarkable growth is possible because of advancements in AI technologies. These advancements in AI technologies empower vehicles to navigate intricate and challenging environments gracefully. They enable these vehicles to make instantaneous, context-aware decisions, ensuring heightened safety and efficiency in their interactions with other vehicles and the surrounding infrastructure.
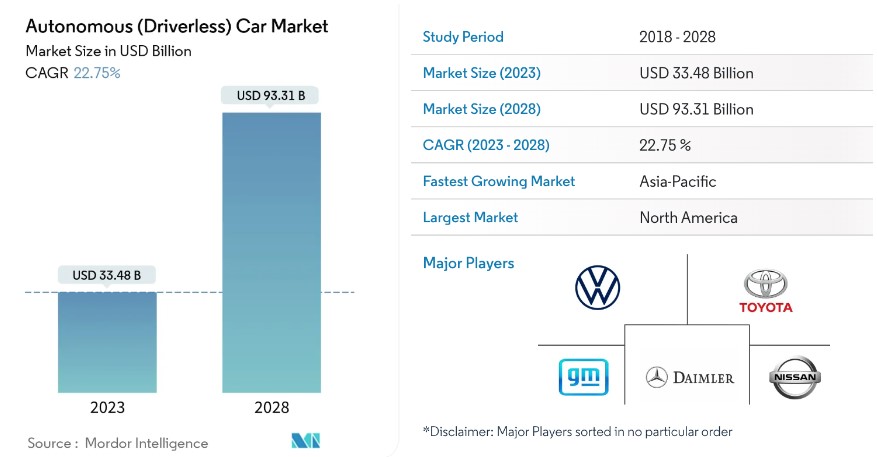
However, the path to fully autonomous vehicles has its challenges. There are ongoing concerns and debates around regulatory frameworks, ethical implications, and cybersecurity risks associated with these AI-driven systems. Despite these challenges, progress is being made. This progress is a result of collaborative efforts. Tech companies, automotive manufacturers, and regulatory bodies are working together. Their goal is a future where autonomous vehicles are commonplace and safe.
As we witness this collaborative innovation restructuring the automotive landscape, it’s essential to understand the underlying technologies. In this article, we’ll explore the different AI techniques used in the autonomous vehicle industry. Let’s dive right into it!
Understanding AI in Autonomous Driving
Integrating AI-related technologies such as computer vision, generative AI, GPU acceleration, and IoT edge computing creates a sophisticated system that makes autonomous driving possible. Computer vision and GPU acceleration facilitate real-time environmental perception, while generative AI simulates scenarios for algorithm refinement and testing. IoT with edge computing ensures seamless communication for safe navigation. This combination empowers autonomous vehicles to navigate complex environments autonomously.
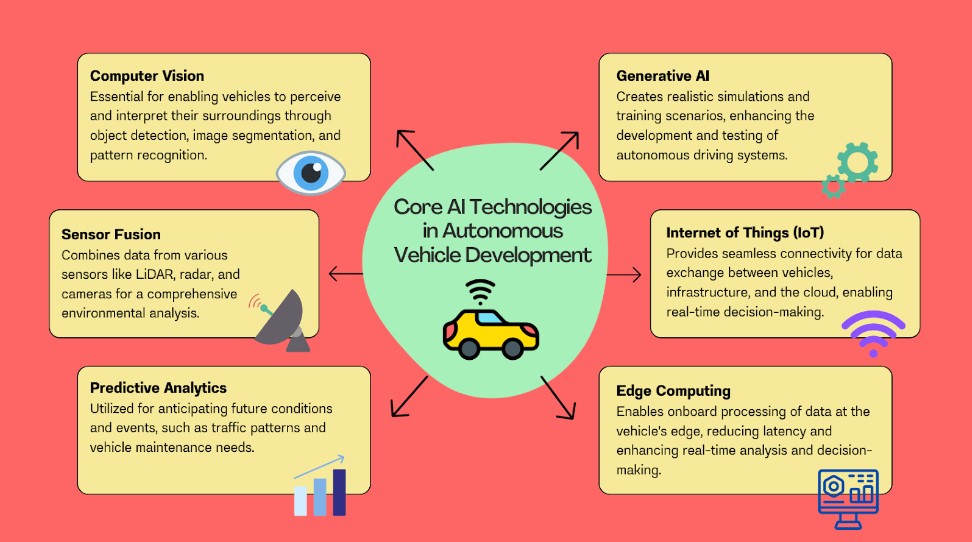
Furthermore, integrating cameras, LiDAR (Light Detection and Ranging), and radar in autonomous vehicles creates a complex 360-degree observation system. This system is essential for ensuring the vehicle’s awareness of its surroundings and enhancing safety. With their advanced imaging capabilities, cameras capture a wide spectrum of visual data from the surrounding environment. LiDAR, or Light Detection and Ranging, is a sophisticated sensor that employs precise laser pulses to measure distances with unparalleled accuracy, crafting intricate 3D terrain maps simultaneously. Radar uses high-frequency radio waves to detect various objects within the vehicle’s vicinity, providing an additional layer of safety and awareness to the autonomous system. Together, they provide a complete view of the vehicle’s environment, enabling it to detect obstacles, pedestrians, and other vehicles from all angles and make informed decisions for safe navigation.
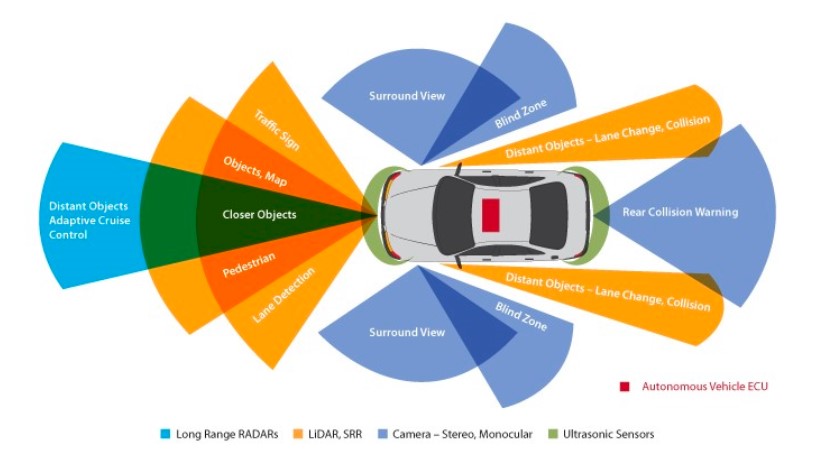
These technological advancements have paved the way for the rapid adoption of autonomous vehicles. Notably, 38% of consumers in the United States are ready to embrace these vehicles. By 2040, it’s projected that more than 33 million autonomous vehicles could be on global roads. This gradual shift signifies a future where autonomous vehicles redefine how we commute daily, reshape transportation infrastructure, and subtly influence societal norms.
Next, we’ll focus on the heart of self-driving cars - autonomous navigation. This pivotal component requires vehicles to create complex choreographies where every move synchronises perfectly with the ever-changing surroundings.
Computer Vision and GPU Acceleration in Autonomous Navigation
Autonomous navigation involves vehicles being able to navigate and operate without human intervention. It’s a complex task that requires the vehicle to perceive its environment, make decisions, and control its operation in real time. Computer vision and GPU acceleration can work together to solve this complex task.
Autonomous vehicles use computer vision systems, enhanced by techniques such as deep neural networks, to perceive and interpret the world around them. Using cameras and other sensors, they gather visual information from the environment, which is then processed to detect and classify objects, recognise patterns, and understand the spatial arrangement of the surroundings. However, the immense computational requirements of processing and analysing the vast amounts of real-time data these sensors capture pose a significant challenge. This is where GPU acceleration becomes vital.
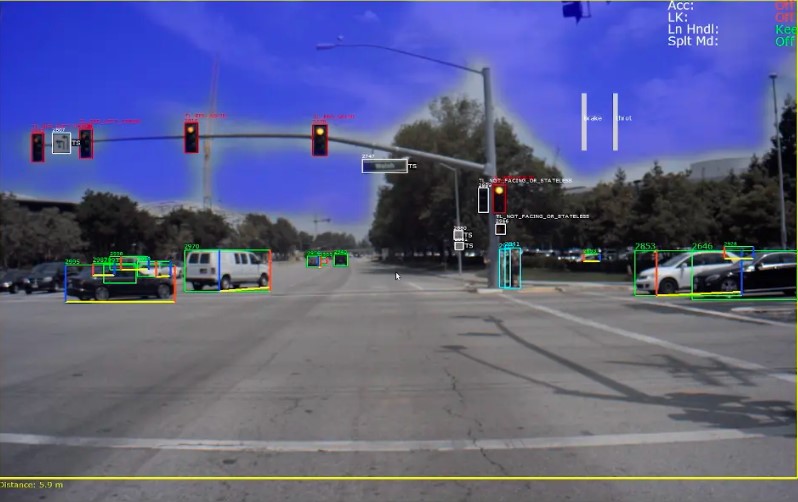
With their parallel processing capabilities, GPUs can handle the extensive computations required by computer vision algorithms much faster than traditional CPUs. This acceleration is critical for real-time processing. This is important because autonomous vehicles must be able to understand dynamic traffic conditions. For instance, if a pedestrian unexpectedly jaywalks across the street, the vehicle must quickly detect and react appropriately to such situations. The capacity of autonomous vehicles to instantly respond to their environment is key to their safe and effective integration into our transportation infrastructure.
Their capacity for instant response must undergo rigorous testing. This testing phase involves exhaustive evaluation to fine-tune the algorithms and technologies that power these vehicles. Only through such thorough testing can the promise of autonomous mobility be realised. However, not all testing scenarios are easy to test; this is where generative AI can step in.
Generative AI for Simulation and Testing
Using generative AI for simulation and testing while developing autonomous vehicles is a relatively new and innovative approach. Generative AI can help create realistic and varied driving scenarios crucial for training and evaluating autonomous driving systems. For example, the model GAIA-1, designed explicitly for autonomy, uses video, text, and action inputs to create highly realistic driving videos. Such detailed simulation helps provide diverse driving experiences, many of which would be rare or challenging to encounter in real-world tests.
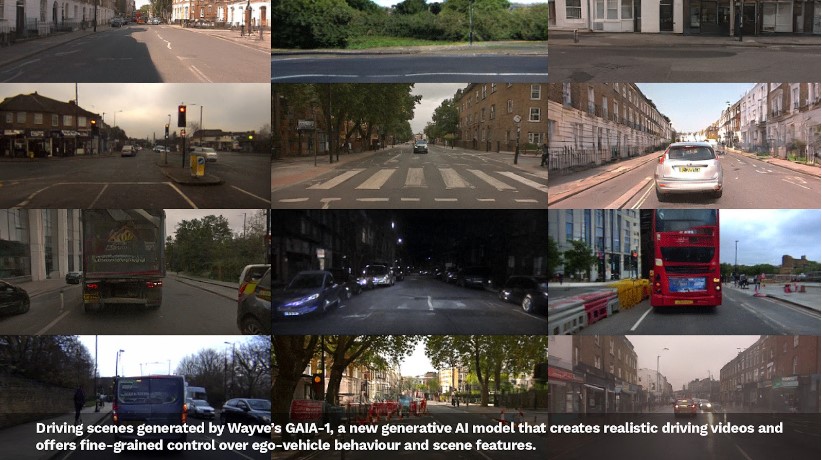
Generative AI can simulate multiple possible futures from a given starting point. For instance, it can show how a self-driving car might react to a pedestrian suddenly crossing the street in different ways. The autonomous car might suddenly hit the brake or swerve to avoid the pedestrian, etc.
This capability of GAIA-1 to generate “what if” scenarios is very valuable. It helps in testing how safe and effective autonomous driving systems are by overcoming the challenges of testing these vehicles in the real world. Some test situations might be too risky or complicated to set up on actual roads.
IoT and Edge Computing in Vehicle Connectivity
On a road where all the cars are driverless, it becomes essential that the cars are connected and able to communicate. Internet of Things (IoT) and edge computing are technologies that can help make vehicle-to-vehicle (V2V) and vehicle-to-infrastructure (V2I) connectivity reliable and simple.
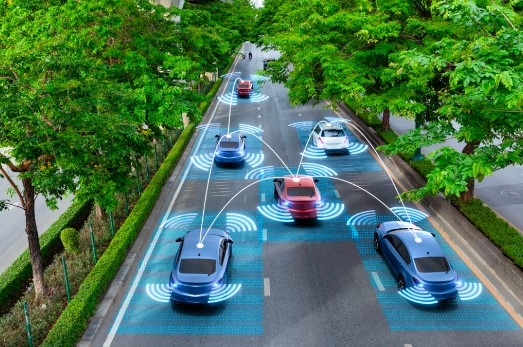
IoT facilitates establishing an interconnected network that allows vehicles to ‘talk’ to each other and to road infrastructure like traffic lights, road signs, and sensors. Then, vehicles can share information about road conditions, traffic congestion, accidents, and weather conditions in real-time. Sharing information enables autonomous cars to make more informed decisions, potentially reducing the likelihood of accidents and improving traffic flow.
In this network, edge computing plays a vital role in processing data locally, near the source of data generation (i.e., the vehicle). This is crucial for low-latency responses in critical situations. In scenarios where split-second decisions can be the difference between safety and collision, the speed at which data is processed and responded to is essential.
Integrating IoT and edge computing into vehicle connectivity has the potential to change how vehicles interact with each other and their surroundings. Coinciding with this technological shift, the economic landscape is also evolving. The market value of IoT products and services for autonomous vehicles is predicted to range from 110 to 130 billion U.S. dollars by 2025. This value is forecasted to soar to between 240 and 300 billion U.S. dollars by 2030, signifying a pivotal expansion of IoT’s influence in the autonomous vehicle industry
Challenges in Autonomous Vehicle AI
Despite the latest advancements in AI innovations, autonomous vehicles have various challenges for manufacturers. Most of these challenges stem from the unpredictable nature of the road. Autonomous cars must navigate unexpected traffic pile-ups, animal crossings, pedestrian movements, potholes, road closures, and construction sites. They must also make quick and accurate decisions to avoid harm to people or property.
Making quick decisions becomes a challenge when there are issues in receiving timely updates. For example, if a vehicle doesn’t receive information about a closed road ahead, it may continue towards it, potentially causing an accident. Autonomous vehicles also need real-time information on environmental conditions. So that driving behaviour can be adjusted in response to weather changes, like reducing speed during rainfall or modifying in-car settings like air conditioning in response to external temperatures.
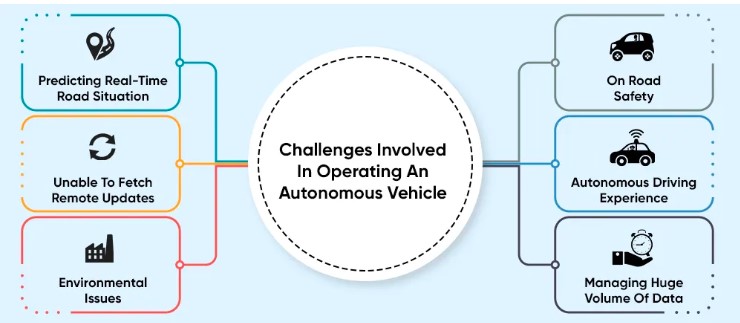
In addition to these challenges, safety and the need for manual intervention also pose significant concerns. Even with advanced technology, most self-driving cars are not entirely autonomous and require some level of human oversight. This affects the driving experience. The experience should be seamless and need no intervention from the passenger.
Also, managing the enormous amounts of data generated by autonomous vehicles is quite a challenge. For instance, a single eight-hour journey can produce up to 100 terabytes of data. Handling this data requires immense storage capacity, efficient processing, and almost perfect uptime to ensure the vehicle operates safely and effectively.
Addressing these challenges requires expertise, and this is where TechnoLynx can step in to help you out. TechnoLynx is a software research and development consultancy specialising in artificial intelligence and computer vision. We focus on creating accurate and efficient AI models to analyse and interpret visual data. We leverage machine learning methods such as deep learning to provide customised solutions tailored to specific client needs.
What We Can Offer as TechnoLynx
At TechnoLynx, we specialise in creating custom AI solutions. We focus on integrating advanced AI technologies into practical applications. Our expertise in AI for computer vision, generative AI, GPU acceleration, and IoT edge computing is pivotal in enhancing the functionality and safety of autonomous vehicles.
We have capabilities in object detection to apply for lane and pedestrian detection. Using tools like PyTorch, TensorFlow, and CUDA, we develop high-performance applications that enable real-time decision-making based on visual data, catering to the complex demands of autonomous vehicle technology. Please contact us for more information or to explore our innovative AI solutions for autonomous vehicles.
Conclusion
The advancements in AI for autonomous vehicles can potentially lead to a significant transformation in transportation. By integrating cutting-edge technologies such as generative AI, computer vision, GPU acceleration, and IoT edge computing, autonomous vehicles are poised to reshape everyday life. However, this transformation presents unique challenges, especially in ensuring safety and managing the substantial data volumes generated.
Tackling these challenges head-on, TechnoLynx can help your business emerge as a leader in the autonomous vehicle space. By leveraging our expertise in AI technologies, we can develop tailored solutions to enhance autonomous vehicles’ functionality and safety. Our specialised AI-driven approaches are designed to address the unique demands and complexities of the autonomous vehicle industry, ensuring a safer and more efficient future for transportation. Contact us to explore how we can help your business lead in this transformative space.
Sources for the images:
Bockenbach, O. (2019) Autonomous Driving and Artificial Intelligence An Approach to Achieve Functional Safety KPIT Technologies.
Cvijetic, N. (2019) Taking It to the Streets: Ride in an NVIDIA Self-Driving Car with DRIVE Labs NVIDIA Corporation.
IWayve Technologies Ltd. (2023) Introducing GAIA-1: A Cutting-Edge Generative AI Model for Autonomy.
Mordor Intelligence Research & Advisory. (2023). Autonomous Vehicle Market Size & Share Analysis - Growth Trends & Forecasts (2023 - 2028).
Recogni (2020) Autonomous Vehicles And A System Of Connected Cars AI Time Journal.
Singh, K. (n.d.) Business Operations On Autopilot: 6 Ways To Overcome The Challenges In Operating Autonomous Vehicles X-Byte Enterprise Solutions.